Intelligent augmented reality application for personalised rhinoplasty using machine learning
Abstract
Rhinoplasty, a common yet complex cosmetic surgery, often results in patient dissatisfaction due to the reliance on subjective surgeon evaluations. This study introduces an intelligent augmented reality (AR) application for personalised nose surgery, integrating three core innovations: (1) Preoperative 3D Modelling; (2) Machine Learning (ML) Analysis; and (3) AR Visualisation. The system employs advanced computer vision algorithms to extract precise facial measurements from high-resolution 3D scans or photographs. These measurements are analysed using ML techniques to calculate key facial ratios and recommend optimal nose shapes tailored to individual facial structures. AR further enhances the surgical process by providing real-time visualisations and guidance, enabling surgeons to implement data-driven decisions with greater precision. This novel approach addresses key challenges in rhinoplasty by automating critical steps of the surgical planning process, reducing subjectivity, and significantly improving surgical accuracy. The application’s contribution extends beyond the operating room, offering surgeons a powerful educational tool with real-time feedback and interactive visualisations to support continuous skill development. This study represents a transformative step in leveraging AR and ML for enhanced precision, patient satisfaction, and surgical outcomes in cosmetic surgery.
Keywords
1. INTRODUCTION
Rhinoplasty, a commonly sought-after cosmetic surgery, consistently ranks among the most popular procedures worldwide. It addresses not only aesthetic desires but also functional concerns [Figure 1]. Despite its popularity, achieving personalised and satisfactory outcomes remains a challenge, as evidenced by a review of 2,326 cases where the overall satisfaction rate was 83.6%[1]. This high level of patient dissatisfaction, especially among males, underscores the complex nature of this surgical endeavour and the need for more tailored and effective solutions.
Figure 1. Rhinoplasty, sometimes known as a “Nose Job”, is a surgical procedure to remodel the nose. The figure shows cartilage and bone removal during rhinoplasty (top) and compares open and closed approaches (bottom). Open rhinoplasty involves an external incision across the columella, while closed rhinoplasty uses internal incisions, leaving no visible scars.
The motivation for this study arises from the desire to enhance patient satisfaction and outcomes in rhinoplasty. Traditional surgical techniques often rely on the surgeon’s experience and intuition, leading to suboptimal results. This study aims to address common concerns such as residual dorsal humps and under-rotated tips, which are significant contributors to patient dissatisfaction [Table 1][2]. By integrating artificial intelligence (AI) and augmented reality (AR) into rhinoplasty, this research seeks to improve the precision and personalisation of the surgery, ultimately boosting patient satisfaction and confidence.
Reasons given by dissatisfied male and female patients in rhinoplasty
Dissatisfied rhinoplasty patients | |||
Males | Females | P value | |
Residual dorsal hump | 46.5% | 69.1% | < 0.001 |
Tip under-rotated | 29.5% | 56.4% | < 0.001 |
Tip bulbous | 21.7% | 46.0% | < 0.001 |
Nose too large | 21.7% | 44.1% | 0.001 |
Nose too small | 24.0% | 39.7% | 0.002 |
Tip pinched | 20.2% | 37.7% | < 0.001 |
Nose deviated | 23.3% | 32.9% | 0.05 |
Expecting life to improve | 23.3% | 29.5% | NS |
Excessive scarring | 17.8% | 34.9% | < 0.001 |
Difficulty breathing | 20.9% | 30.2% | NS |
Poor communication by the surgeon | 13.9% | 23.0% | 0.04 |
Nose too wide | 10.1% | 25.4% | < 0.001 |
Tip over-rotated | 10.1% | 16.3% | NS |
Look like a different person | 7.0% | 19.4% | 0.001 |
Look like a different ethnicity | 3.1% | 3.6% | NS |
Traditional rhinoplasty methods primarily depend on the surgeon’s subjective evaluations, which can result in inconsistent outcomes depending on the patient’s motivations, as stated in Table 2[2]. These techniques include manual preoperative planning and intraoperative adjustments based on the surgeon’s experience. The proposed method in this study involves the development of an intelligent AR application that utilises preoperative 3D modelling, machine learning (ML) algorithms to find the best nose recommendation for each patient, and real-time AR visualisation. This innovative approach provides surgeons with precise, data-driven guidance during the procedure, aiming to optimise surgical strategies and improve outcomes.
Motivation for rhinoplasty given by satisfied male and female (Khansa et al., 2016[2])
Satisfied rhinoplasty patients | ||
Males | Females | |
Prior nasal fracture | 15.2% | 16.5% |
Dorsum deviated | 12.7% | 13.5% |
The nose was too large | 3.0% | 3.8% |
The nose was too wide | 1.8% | 2.1% |
The nose was too small | 0.6% | 1.7% |
Tip was under-rotated | 0.6% | 0.5% |
The nose was too narrow | 0.6% | 0.2% |
Tip was over-rotated | 0% | 0.7% |
1.1. Novelty and contribution
This research introduces a novel intelligent AR application for personalised nose surgery, which integrates three innovative components:
Preoperative 3D Modelling: Leveraging advanced computer vision algorithms to capture and analyse detailed facial measurements from high-resolution 3D scans or photographs.
ML Algorithms: Calculating key facial ratios and making data-driven recommendations for optimal nose shapes tailored to individual facial structures.
AR Visualisation: Providing surgeons with real-time, data-driven guidance during surgery, enabling precise implementation of the surgical plan.
The key technical difficulty addressed in this study is the challenge of maintaining accuracy and reliability across the multiple stages of data processing - capturing, analysing, and visualising patient-specific facial features. This study overcomes this challenge by implementing robust data preprocessing techniques, state-of-the-art landmark detection algorithms, and seamless integration of AR for real-time surgical support.
1.2. Significance
The proposed intelligent AR application addresses limitations in traditional methods by automating critical steps in the surgical planning process, which were previously dependent on subjective judgment. This automation significantly reduces variability and enhances precision, resulting in improved surgical outcomes. Furthermore, the application serves as an invaluable educational tool, equipping surgeons with real-time feedback and interactive visualisations that accelerate learning and skill development.
The primary outcome of this research is the development of an intelligent AR App for personalised nose surgery. This app is expected to significantly enhance the precision of rhinoplasty procedures, reduce complications, and improve patient satisfaction. Moreover, it serves as an invaluable educational tool for aspiring surgeons, potentially reducing the learning curve for complex surgical decisions.
1.3. Problem statement
Rhinoplasty, despite being one of the most performed and requested cosmetic surgeries worldwide, presents significant challenges in achieving satisfactory outcomes. The nose’s prominent position on the face means that even minor discrepancies can lead to noticeable and often disappointing results. Patient dissatisfaction rates, as noted in various studies, highlight the complexity and high expectations associated with this procedure. A primary issue in rhinoplasty is the reliance on the surgeon’s subjective judgment and experience, which can lead to variability in outcomes. Traditional methods often depend on two-dimensional (2D) imaging and manual adjustments, which do not fully capture the intricacies of individual nasal anatomy[3]. This lack of precision can result in suboptimal aesthetic and functional results, necessitating secondary surgeries and further interventions[4]. Nasal asymmetry is a common challenge in rhinoplasty. Achieving perfect balance during surgery is difficult due to natural variations in nasal bones and cartilage, and the healing process can further impact symmetry[4]. This complexity underscores the need for more advanced preoperative planning and intraoperative guidance tools. The psychological impact of unsatisfactory rhinoplasty outcomes is significant. Patients often experience anxiety, depression, and reduced self-esteem when their cosmetic goals are not met[4]. Moreover, the societal pressure to conform to certain beauty standards exacerbates these psychological effects, making the pursuit of perfection in rhinoplasty even more critical.
1.4. Literature review
The field of rhinoplasty has evolved significantly over the past decades, with advancements in surgical techniques and medical technologies aiming to improve patient outcomes and satisfaction. Despite these advancements, achieving consistent and satisfactory results remains a challenge due to the complex nature of nasal anatomy and the reliance on the surgeon’s subjective judgment. Rhinoplasty has a long history, tracing back to ancient civilisations where nasal reconstruction was practised to restore form and function. Early techniques were primarily reconstructive, addressing severe disfigurements often resulting from punishments or injuries. Notably, Sushruta, an ancient Indian surgeon, is credited with pioneering rhinoplasty techniques around 600 BCE[5]. This historical context laid the foundation for modern rhinoplasty practices. Modern rhinoplasty techniques have become increasingly sophisticated, incorporating both open and closed approaches to address a wide range of aesthetic and functional concerns. Preservation rhinoplasty, for example, emphasises maintaining as much of the nasal structure as possible while achieving the desired aesthetic goals[6]. Advances in ultrasound technology have further enhanced the precision of these procedures, allowing for more accurate planning and postoperative evaluations[6]. Achieving nasal symmetry is one of the major challenges in rhinoplasty. Many patients present with pre-existing asymmetries, complicating the surgical process. Techniques such as osteotomies, cartilage grafts, and septal corrections aim to enhance symmetry, but complete balance is often difficult to achieve due to natural variations in the nasal structure and the healing process[4]. Furthermore, the presence of scar tissue and structural changes from previous surgeries add complexity to revision procedures. The psychological impact of rhinoplasty on patients is profound. Studies have shown that dissatisfaction with surgical outcomes can lead to anxiety, depression, and reduced self-esteem[5]. The societal pressure to meet certain beauty standards exacerbates these psychological effects, making the need for precise and satisfactory surgical outcomes even more critical. The integration of advanced technologies such as 3D modelling, ML, and AR into rhinoplasty has the potential to address many of the existing challenges. Preoperative 3D modelling allows for a more accurate understanding of the patient’s unique nasal anatomy[6], enabling surgeons to formulate precise surgical plans[7]. ML algorithms can analyse these models to provide data-driven recommendations based on the patient’s facial features, enhancing the surgeon’s ability to achieve optimal outcomes[8]. AR technology, which superimposes computer-generated imagery onto the real-world view, has shown promise in enhancing surgical precision. AR applications in medicine range from medical education and training to intraoperative guidance. In rhinoplasty, AR can provide real-time guidance, overlaying digital information onto the surgical field to assist surgeons in executing precise surgical plans[9]. This technology has the potential to reduce surgical errors and improve patient outcomes significantly. While current technologies such as 2D imaging and manual surgical planning offer some benefits, they fall short of providing the comprehensive insights needed for optimal rhinoplasty outcomes and nose match-finding techniques. The transition to advanced technologies such as 3D modelling, ML algorithms, and utilising AR aims to overcome these limitations by offering more detailed and interactive preoperative and intraoperative tools[9]. The literature indicates that while rhinoplasty has advanced significantly, there are still substantial challenges that need to be addressed to achieve consistent and satisfactory outcomes. The integration of advanced technologies presents a promising solution to these challenges, offering the potential to enhance surgical precision, reduce complications, and improve patient satisfaction. This study aims to contribute to this evolving field by developing an intelligent AR App for personalised nose surgery, leveraging these technologies to set new standards in rhinoplasty.
1.5. Gaps and limitations in current technology
Despite significant advancements in rhinoplasty techniques and the integration of technology, several gaps and limitations persist in current methodologies. Existing tools and approaches often fail to fully address the need for personalised surgical planning and real-time intraoperative guidance, both of which are critical for enhancing outcomes and patient satisfaction.
1.5.1. Limited personalisation
While 3D modelling and computer-aided planning provide valuable visualisations, they often lack the precision needed to customise procedures to the unique anatomical features of individual patients. Current systems do not fully integrate patient-specific data into surgical planning, leaving room for variability in outcomes.
1.5.2. Lack of real-time feedback
Many existing technologies focus solely on preoperative planning and provide minimal support during surgery. Surgeons often rely on their expertise and intuition during procedures, which can lead to inconsistent results.
1.5.3. Underutilisation of AR
While AR has been explored in various medical fields, its application in rhinoplasty remains limited. Current implementations are primarily experimental, and their potential for real-time guidance and improved communication between surgeons and patients has not been fully realised.
1.5.4. Insufficient integration with AI
Although AI has been successfully applied in areas such as diagnostics and imaging, its role in optimising surgical outcomes in rhinoplasty has yet to be comprehensively explored. Current systems lack the ability to predict outcomes based on historical data or to adapt surgical plans dynamically.
1.6. Novelty of this study
This study addresses these limitations by integrating AR and ML into rhinoplasty, offering several innovative contributions:
Enhanced Personalisation: By leveraging AI-driven preoperative 3D modelling, this study provides an advanced level of customisation, enabling surgical plans tailored to the patient’s unique nasal anatomy and aesthetic preferences.
Real-Time Surgical Guidance: The developed application employs AR technology to offer surgeons real-time visual overlays, improving precision during critical surgical steps and reducing reliance on intuition.
Predictive Capabilities with AI: ML algorithms analyse historical surgical data to predict optimal outcomes, enabling more informed decision-making and reducing the likelihood of complications or revisions.
Improved Patient-Surgeon Communication: The use of realistic 3D previews generated through AR allows patients to visualise potential outcomes, setting realistic expectations and fostering better communication.
Educational Value: The application doubles as a training tool for aspiring surgeons, offering simulated scenarios that mimic real-life procedures, thereby enhancing surgical education and preparedness.
By addressing the gaps in current technologies and introducing these novel contributions, this study paves the way for a transformative approach to rhinoplasty, setting a new standard for precision, personalisation, and patient satisfaction in cosmetic surgery.
2. METHODS
This study aims to develop an intelligent AR App for personalised nose surgery (Rhinoplasty) that uses advanced 3D modelling, ML algorithms, and real-time AR visualisation. This application seeks to enhance surgical precision, reduce postoperative complications, and significantly improve patient satisfaction by providing surgeons with precise, data-driven guidance and patients with realistic previews of their postoperative appearance. Through the integration of these cutting-edge technologies, the project aspires to revolutionise the field of rhinoplasty, setting new standards for surgical accuracy and patient care. The methodology of this research integrates both theoretical and practical approaches to explore the use of AR and ML in rhinoplasty. The research employs a mixed-methods approach, combining qualitative analysis of AR and 3D facial reconstruction technologies with quantitative analysis of market adoption and technological trends [Figure 2].
Figure 2. This diagram outlines the four-phase development process of the AR-based system. The figure is an original creation specifically designed for this study. Phase 1 (Requirement Analysis) focuses on understanding user needs, gathering requirements, and evaluating technical feasibility; Phase 2 (Design) involves architectural planning and UI design; Phase 3 (Implementation) covers application development, integration of third-party APIs, and testing; and Phase 4 (Testing) ensures functionality, user feedback incorporation, and bug resolution to finalise the application. AR: Augmented reality; UI: user interface; APIs: application programming interfaces.
This study employs a systematic approach to develop and validate a personalised nose recommendation system using AR and ML. The methodology integrates both qualitative and quantitative techniques to ensure a comprehensive evaluation of the system’s design and functionality. The research adopts a primarily quantitative approach supported by qualitative analysis where necessary. This mixed-methods strategy enables a detailed exploration of the technological capabilities and limitations while providing statistical insights into the effectiveness of the proposed system. This section describes the methodology employed in designing a personalised nose recommendation system, integrating advanced facial measurement techniques, ML algorithms, and AR. The system is designed to provide customised nose shape recommendations through a series of stages, from data collection to 3D model generation and AR integration.
2.1. Data collection and feature analysis
2.1.1. Participant information
The study included a single participant, a healthy 40-year-old Asian female with no prior history of nasal surgery or facial trauma. The participant was selected based on the following criteria:
Inclusion Criteria: Individuals with no prior nasal surgery or trauma to ensure baseline facial measurements were unaffected by previous medical interventions.
Exclusion Criteria: Individuals with prior nasal surgeries, significant facial asymmetry, or medical conditions affecting nasal anatomy were excluded from consideration.
2.1.2. Data collection
Facial measurements and anatomical features were captured using high-resolution 3D imaging systems to ensure precise modelling. These measurements were analysed to calculate nasal dimensions and proportions, which were then used to generate recommendations for a new nose design. The designed nose was integrated into the AR system to visualise the predicted post-surgery outcomes.
The initial step in the system design involves collecting detailed facial measurements. High-resolution 3D scans or photographs of the patient’s face are captured using advanced imaging tools. These measurements are essential for creating an accurate 3D model of the patient’s face [Figures 3 and 4]. Key facial measurements include facial width (FW, distance between cheekbones), facial height (FH, distance from hairline to chin), intercanthal distance (ID, distance between inner eye corners), nasal width (width of the nose at the widest point), nasal length (NL, distance from nasion to nasal tip), nasal bridge width (NBW, width at the narrowest point of the nasal bridge), alar base width (ABW, distance between outer edges of nostrils), tip projection (TP, distance the nasal tip projects from the face), nasal tip angle, lip-to-nose distance (LND, distance from the base of the nose to the upper lip), and chin projection (CP) [Figure 5].
Figure 3. Area measurements: from left to right top row: Alar base, Dorsal hump, Entire nose. Bottom row: Nasal Dorsum, Tip. This figure is quoted with permission from Celikoyar, Topsakal, and Sawyer, 2023[10].
Figure 4. Volume measurements: from left to right top row: Alar base, Dorsal hump, Entire nose. Bottom row: Nasal Dorsum, Tip. This figure is quoted with permission from Celikoyar, Topsakal, and Sawyer, 2023[10].
Figure 5. The image highlights the critical facial features and measurements used in the personalised nose recommendation system. Green lines illustrate FW, FH, ID, nasal width, NL, NBW, ABW, TP, nasal tip angle, LND, and CP. These measurements are essential for creating accurate 3D models and personalised nose shape recommendations. FW: Facial width; FH: facial height; ID: intercanthal distance; NL: nasal length; NBW: nasal bridge width; ABW: alar base width; TP: tip projection; LND: lip-to-nose distance; CP: chin projection.
Once the facial measurements are collected, key facial ratios are calculated to analyse the proportions and symmetry of the face. These ratios provide a standardised way to compare different facial features and ensure the recommendations are personalised. The key facial ratios include the width-to-height ratio (RWH), intercanthal-to-facial width ratio (RIFW), nasal length-to-facial height ratio (RNLH), nasal bridge width-to-facial width ratio (RNBW), alar base width-to-facial width ratio (RABW), tip projection-to-facial height ratio
2.2. Technical approach
The nose recommendation algorithm uses these calculated facial ratios to suggest an optimal nose shape. The algorithm considers predefined nose types, each with specific dimensions. The recommendation function evaluates the facial ratios and selects the nose type that best matches the patient’s facial proportions. For instance, a particular nose type might be recommended if the RWH is below a certain threshold and the RNBW is within a specific range.
The RWH is calculated by dividing the FW by the FH, as given in
This ratio helps determine the proportional relationship between the width and height of the face, providing a basis for personalised nose shape recommendations that harmonise with the patient’s overall facial structure.
The RIFW is calculated by dividing the ID by the FW, as given in
This ratio assesses the spacing between the inner corners of the eyes in relation to the overall width of the face, aiding in the recommendation of a nose shape that complements the patient’s eye spacing and facial proportions.
The RNLH is calculated by dividing the NL by the FH, as given in
This ratio provides insight into how the length of the nose relates to the overall height of the face, helping to recommend a nose shape that is proportionate to the patient’s facial dimensions.
The RNBW is calculated by dividing the NBW by the FW, as given in
This ratio evaluates how the width of the nasal bridge compares to the overall width of the face, ensuring that the recommended nose shape maintains harmonious facial proportions.
The RABW is calculated by dividing the ABW by the FW, as given in
This ratio assesses the width of the nostrils’ base in relation to the overall FW, aiding in recommending a nose shape that fits well with the patient’s facial proportions.
The RTP is calculated by dividing the TP by the FH, as given by
This ratio helps to evaluate how far the nasal tip projects in relation to the overall height of the face, ensuring the recommended nose shape is balanced and proportional to the patient’s facial dimensions.
The RLND is calculated by dividing the LND by the FH.
This ratio helps to assess how the distance between the base of the nose and the upper lip compares to the overall height of the face, which is important for recommending a nose shape that harmonises with the patient’s facial proportion.
The RCP is calculated by dividing the CP by the FH.
This ratio evaluates how the projection of the chin relates to the overall height of the face, ensuring that the recommended nose shape is balanced and proportionate to the patient’s facial features.
2.2.1. The slope of the nose calculation
To calculate the slope of the nose (Snose), we need to consider the NL, the TP, and the height of the nose bridge (NBH), which is derived from the nasion (midpoint between the eyes where the nasal bridge starts) to the NBW, as given by
These calculations provide an additional dimension to the nose recommendation system, ensuring the Snose is also considered when recommending the optimal nose shape.
After recommending an initial nose shape, the system allows for further customisation based on patient and surgeon preferences. Adjustments can be made to the recommended nose model, such as changes in TP or nasal width. This step ensures that the final nose model meets both aesthetic goals and practical considerations.
The following pseudo-code in Algorithm 1, outlines an advanced algorithmic approach for personalised rhinoplasty, integrating computer vision techniques and ML principles. This algorithm utilises facial landmark detection, feature extraction, and ratio calculations to recommend an ideal nose shape based on the patient’s unique facial dimensions. By analysing key ratios such as the RWH and RNBW, the algorithm identifies the most suitable nose type from predefined templates. Furthermore, the system allows for customisation according to patient and surgeon preferences, ensuring the final nose model meets both aesthetic goals and anatomical considerations.
Algorithm 1: Personalised Rhinoplasty using AR
# Step 1: Data Collection and Preprocessing
def preprocess_images(images):
preprocessed_images = [ ]
for image in images:
# Apply noise reduction, histogram equalisation, and normalisation
processed_image = apply_preprocessing(image)
# Detect and crop facial regions using a face detection algorithm
cropped_face = detect_and_crop_face(processed_image)
preprocessed_images.append(cropped_face)
return preprocessed_images
# Step 2: Facial Landmark Detection
def detect_facial_landmarks(images):
facial_landmarks = [ ]
for image in images:
landmarks = detect_landmarks(image) # Use a pre-trained CNN for landmark detection
facial_landmarks.append(landmarks)
return facial_landmarks
# Step 3: Feature Extraction and Ratio Calculation
def calculate_facial_ratios(landmarks):
ratios = {}
for landmarks in facial_landmarks:
# Extract facial measurements from landmarks
FW = distance_between_cheekbones(landmarks)
FH = distance_from_hairline_to_chin(landmarks)
ID = intercanthal_distance(landmarks)
NL = nasal_length(landmarks)
NBW = nasal_bridge_width(landmarks)
ABW = alar_base_width(landmarks)
TP = tip_projection(landmarks)
LND = lip_to_nose_distance(landmarks)
CP = chin_projection(landmarks)
# Calculate facial ratios
RWH =
RIFW =
RNLH =
RNBW =
RABW =
RTP =
RLND =
RCP =
# Calculate the slope of the nose
NBH =
Snose =
ratios[landmarks] = {
'RWH': RWH, 'RIFW': RIFW, 'RNLH': RNLH, 'RNBW': RNBW,
'RABW': RABW, 'RTP': RTP, 'RLND': RLND, 'RCP': RCP,
'Snose': Snose
}
return ratios
# Step 4: Nose Shape Recommendation
def recommend_nose_shape(ratios):
predefined_nose_types = load_predefined_nose_types()
best_match = None
best_match_score = float('inf')
for nose_type in predefined_nose_types:
score = evaluate_nose_type(nose_type, ratios)
if score < best_match_score:
best_match = nose_type
best_match_score = score
return best_match
def evaluate_nose_type(nose_type, ratios):
score = 0
# Evaluate ratios against predefined nose type
if ratios['RWH'] < nose_type['RWH_threshold']:
score += 1
if nose_type['RNBW_min']
score += 1
# Add evaluations for other ratios as needed
return score
# Step 5: Customisation and Visualisation
def customise_nose_shape(nose_shape, preferences):
customised_nose = nose_shape.copy()
# Apply adjustments based on patient and surgeon preferences
customised_nose['tip_projection'] += preferences['tip_projection_adjustment']
# Other adjustments as necessary
return customised_nose
2.2.2. Nose recommendation function
The nose recommendation algorithm uses the calculated facial ratios to suggest an optimal nose shape. The algorithm considers predefined nose types, NA, NB, NC, ND, and NE, each with specific dimensions. The recommendation function fnose is defined as
Where:
This function evaluates the facial ratios and selects the nose type that best matches the patient’s facial proportions.
2.2.3. Customisation and adjustment
After recommending an initial nose shape, the system allows for further customisation based on patient and surgeon preferences. Adjustments can be made to the recommended nose model Nr using a set of modifications, as given in
Where ai represents specific adjustments, such as changes in tip projection or nasal width. This step ensures that the final nose model Na meets both aesthetic goals and practical considerations.
The adjusted nose model is then used to generate a 3D model. This 3D model can be printed or visualised using AR. The AR integration allows the surgeon and patient to interact with the model in real time, assessing its fit and appearance on the patient’s face. This step provides a dynamic and interactive way to evaluate the proposed surgical outcomes and make any necessary adjustments before the procedure.In summary, the methodology involves a detailed process of data collection, feature analysis, algorithmic recommendation, customisation, and AR integration. By leveraging advanced technologies and precise facial measurements, the system provides personalised and accurate nose shape recommendations, enhancing both patient satisfaction and surgical outcomes.
2.4. Computational complexity
The computational complexity of the proposed algorithm was analysed to evaluate its efficiency and scalability. The analysis is based on the following steps [Table 3].
Time and space complexity analysis of the proposed algorithm
Step | Time complexity | Space complexity |
Image preprocessing | O(n·p) | O(n·p) |
Facial landmark detection | O(n·f) | O(n·l) |
Ratio calculations | O(n) | O(n·r) |
Nose shape recommendation | O(m·r) | O(m) |
Customisation and visualisation | O(1) | O(1) |
3. RESULT
The design section outlines the system architecture for integrating ML and AR technology into rhinoplasty procedures. The primary goal is to enable the capture of a patient’s facial structure in 3D using Microsoft HoloLens 2 and to create a personalised surgical plan that can be reviewed and adjusted in real time by both patients and surgeons.
3.1. System architecture
The system architecture leverages 3D capturing technology for capturing and modifying 3D facial images. This architecture aims to bridge the gap between advanced imaging technologies and clinical applications, enhancing patient engagement and surgical precision.
3.2. Components
The architecture includes several core components:
Hardware devices such as the AR headset and a high-performance computing workstation.
Specialised software applications for 3D image capture, editing, and AR visualisation.
Robust data management protocols to ensure security and compliance with medical data regulations.
3.3. Workflow
The workflow is designed to be highly interactive, with user interfaces (UIs) tailored to both surgeons and patients. This ensures comprehensive tools for detailed surgical planning for surgeons and intuitive visualisation of expected outcomes for patients.
3.4. Technology utilisation
Initially, the plan to use HoloLens 2 for detailed 3D facial image capture was reconsidered due to its limitations. Instead, the iOS application Polycam was adopted for its capability to produce detailed 3D images through photogrammetry [Figure 6]. The captured images are processed and utilised for pre-surgical planning and real-time surgical guidance.
3.5. Data security
Ensuring data security is paramount, with measures including data encryption, secure transmission channels, and strict access controls. Compliance with regulations such as GDPR and HIPAA is maintained through continuous monitoring and response strategies.
4. DISCUSSION
4.1. Quantitative impact and future directions
This study demonstrated the feasibility of an AR-based system for enhancing surgical precision in rhinoplasty. While statistical analysis could not be performed due to the study’s single-participant design, the system’s ability to accurately model the participant’s nasal anatomy and provide real-time visual overlays indicates its potential utility. Future research with larger sample sizes will enable quantitative comparisons of surgical outcomes, providing the statistical evidence needed to validate the system’s impact on precision and patient satisfaction.
The study demonstrated significant potential in enhancing patient understanding and imagination of rhinoplasty outcomes through the use of advanced technologies to create realistic 3D sculptures of patients’ faces.
First, the system successfully created highly realistic 3D models of patients’ faces using photographs or scans. This provided patients with a clear and tangible visualisation of potential surgical outcomes. The application utilised measurements of facial dimensions and symmetry to generate accurate 3D models, ensuring that the visualisations were precise and representative of the patient’s unique facial features.
ML algorithms were employed to analyse the facial data and recommend nose styles and sizes that best matched the patient’s current features. This data-driven approach offered a range of 3D model recommendations tailored to the patient’s anatomy. The recommended 3D models could be further refined using Cinema 4D [Figures 7 and 8], allowing for customisation based on patient preferences and surgeon expertise. This interactive process enabled both parties to experiment with different nose styles and sizes in real time.
Figure 7. The raw 3D sculpture produced by Polycam needs to be imported into a 3D editing program such as Cinema 4D, as it requires texturing and colouring to enhance its realism and visual appeal.
Figure 8. 3D model after cropping before the application of texture, various adjustments, and lighting effects using Cinema 4D. These enhancements bring a more realistic and detailed representation to the model, crucial for accurate surgical visualisation and planning.
Cinema 4D provided advanced editing tools that facilitated detailed adjustments to the 3D models
Figure 9. The 3D model is imported into Cinema 4D, where necessary filters and lighting are applied, along with realistic textures, to enhance the model’s appearance from different angles.
Figure 10. The left image shows the current 3D sculpture of the patient’s face before applying the new nose, while the right image displays the edited version with the new nose, adjusted by the surgeon using the recommended size and symmetry provided by the algorithms.
Figure 11. The 3D edited model of the patient’s face, featuring the desired nose size and anatomy, is imported into the HoloLens STK. This allows for pre-surgery visualisation and comparison with the patient’s current nose, providing the patient with a clearer understanding of their anticipated post-surgery appearance.
Figure 12. The image on the left shows the side view of the 3D model of the patient’s nose, whereas the image on the right displays the patient’s face with the new nose, matched with the other features of her face as produced by the nose recommendation algorithm.
The integration with Microsoft HoloLens enabled the import of the 3D models into an AR environment. Surgeons could use hand gestures and realistic live controls to overlay the 3D models onto the patient’s face, providing a dynamic and interactive way to assess the suitability of the proposed changes. During surgery, the overlaid models served as real-time guides, enhancing surgical precision. Surgeons could reference the AR models to ensure that the actual surgical outcomes closely matched the pre-surgical plans.
The comprehensive system improved surgical planning and execution by offering detailed, customisable, and interactive visualisations. This led to higher patient satisfaction as patients had a better understanding of the expected outcomes and could participate actively in the planning process. Surgeons benefited from enhanced intraoperative guidance, reducing the likelihood of revisions and achieving better alignment with the planned outcomes.
Overall, the study’s results indicate that the use of AR, ML, and 3D modelling technologies in rhinoplasty significantly enhances both patient engagement and surgical precision. These preliminary findings suggest a promising future for the integration of these technologies in personalised cosmetic surgery planning and execution.
4.2. Technological shifts and adaptations
Several strategic shifts were necessary to address technical limitations:
Initial Misconceptions with HoloLens: The plan to use Microsoft HoloLens for detailed 3D facial image capture was reconsidered due to its limitations in medical-grade imaging. Instead, the iOS application Polycam was adopted for its capability to produce detailed 3D images through photogrammetry.
Adopting Polycam: Despite not being the initial choice, Polycam proved reliable for 3D facial scanning. Standard operating procedures were developed to ensure high-quality scans, including guidelines on optimal lighting conditions, camera handling, and photo capture techniques.
4.3. Challenges and solutions
Several challenges were encountered, and solutions were implemented to ensure the system’s success. Maintaining the integrity of 3D data as it moved from Polycam to Cinema 4D, and then to the HoloLens, was critical. Rigorous testing protocols and the use of standardised file formats and transfer protocols helped minimise data degradation or corruption. Training users to interact with new technologies was identified as a potential usability challenge. To address this, structured training programs were proposed, including hands-on workshops and video tutorials to familiarise users with the system. Additionally, interactive tutorials were incorporated into the application, providing step-by-step guidance to enhance user proficiency. Finally, feedback loops were implemented, allowing users to report challenges or suggest improvements, enabling continuous refinement of the system to meet user needs and expectations.
The integration of AR and ML in rhinoplasty has shown promising results in enhancing surgical precision and patient satisfaction. The ability to create detailed 3D models and provide real-time visualisations has transformed the surgical planning and execution process, offering a more personalised and precise approach to nasal surgery. The project’s adaptability and continuous learning approach were crucial in overcoming technical challenges and ensuring the system’s effectiveness in a clinical setting.
5. EVALUATION
The integration of ML and AR in rhinoplasty represents a significant advancement in preoperative planning and intraoperative guidance. This technology provides a transformative approach to visualising surgical outcomes, which was the primary goal of this project.
5.1. Comparison with existing technologies
Current Standard Methods: Traditional methods rely heavily on 2D imaging for preoperative planning[11]. These methods involve measurements and assessments based on photographs, which lack the depth and precision necessary for accurate surgical planning.
Existing 3D Simulation Programs: Several 3D simulation programs, such as 3dMDFace and Canfield Vectra, provide advanced visualisation capabilities. However, these systems often struggle with flexibility and precision, leading to disparities between simulated and actual surgical outcomes.
5.2. Reflection on project objectives and outcomes
This project aimed to develop an AR application for personalised nose surgery, initially envisaging the use of AI and ML to recommend optimal nose shapes. While the fully integrated application interface and environment were not developed, significant milestones were achieved. We successfully implemented ML algorithms to recommend nose shapes and utilised 3D visualisation techniques to create realistic models. Additionally, AR was employed to provide a live and realistic 3D plan for the surgery, enhancing surgical planning and patient engagement. These advancements facilitated better decision-making and improved patient satisfaction, achieving the primary objective of the project.
5.3. Evaluation of system efficacy
5.3.1. Practicality of the implemented system
The implemented AR system significantly enhances patient and surgeon experiences through its interactive 3D visualisations. For patients, it improves decision-making, reduces anxiety, and sets realistic expectations, leading to higher satisfaction. For surgeons, it offers improved preoperative planning and intraoperative precision, which are crucial for optimal surgical outcomes[12].
5.3.2. Challenges and strategic shifts
Initial misconceptions about the capabilities of Microsoft HoloLens necessitated a strategic pivot to Polycam for 3D facial scanning. This shift highlighted the importance of rigorous pre-implementation testing in medical technology projects. Polycam proved effective, although dependent on optimal conditions, necessitating the development of standard operating procedures to ensure high-quality scans.
5.4. Technical challenges and adaptation
The project’s adaptive nature was demonstrated by the successful integration of Polycam after realising Hololens’ limitations. Ensuring accurate 3D model rendering required careful control of photo capture conditions, emphasising the need for adaptability and continuous learning in technological innovation.
Compared to traditional methods, the system’s collaborative and interactive elements significantly enhance the consultation process. The ability to present tangible 3D models fosters trust and informed decision-making between surgeons and patients, improving overall treatment outcomes.
5.5. Advantages and limitations
The sophisticated editing capabilities of Cinema 4D surpass traditional 2D editing software, although the need to switch between platforms indicates a potential area for improvement. Future development should focus on creating an integrated, single-platform solution with a robust UI to streamline the process.
6. CONCLUSION
The study successfully explores the transformative potential of ML and AR in personalised nose surgery, specifically rhinoplasty. Utilising advanced technologies such as Polycam for 3D facial scanning, Cinema 4D for precise model manipulation, and Microsoft HoloLens for immersive visualisation, the project aimed to enhance surgical planning and patient engagement. Despite initial challenges, including the incorrect assumption regarding the capabilities of Microsoft HoloLens and the necessary pivot to Polycam, the developed system successfully provided realistic 3D visualisations for both surgeons and patients. This dual benefit improved surgical precision and patient satisfaction. The project’s innovative workflow demonstrated the practicality of AR in medical settings and suggested broader applications for other cosmetic and reconstructive surgeries. Future research should focus on integrating ML for automated facial analysis and developing a consolidated platform for scanning and visualisation. Overall, the dissertation highlights AR’s significant promise in advancing rhinoplasty, improving clinical outcomes, and setting the stage for future technological innovations in healthcare.
The future of AR in rhinoplasty is poised for significant advancements, focusing on the development of a comprehensive AR application that integrates 3D facial scanning, sophisticated editing tools, and real-time visualisation. This all-in-one application aims to streamline the pre-surgical process, enhancing decision-making and patient satisfaction.
The envisioned AR application promises to revolutionise surgical planning and execution in cosmetic and reconstructive surgery, improving clinical outcomes and streamlining workflows for surgeons.
DECLARATIONS
Authors’ contributions
Collected the data, conducted the research, designed and implemented the algorithms, and integrated AR and ML technologies; drafted and revised the manuscript: Heydari, M. S.
Revised the manuscript and provided medical advice: Kolivand, M.
Provided revision, suggestions, and medical advice: Al-Azzawi, M.
Supervised the study and provided administrative and technical support: Kolivand, H.
Availability of data and materials
The source of the raw data is the participant’s 3D facial scan, which was obtained using the Photogrammetry method via the Polycam app. The 3D reconstruction and mesh were created using Cinema 4D, and the authors confirm that they have complied with all copyright regulations of these software applications. Some raw data that support the findings of this study are available from the corresponding author upon reasonable request.
Financial support and sponsorship
None.
Conflicts of interest
All authors declared that there are no conflicts of interest.
Ethical approval and consent to participate
This study did not require ethical approval as it involved data from a single healthy adult participant, and no clinical intervention or trial was conducted. The study’s primary aim was to demonstrate the technical feasibility of the machine learning algorithm in calculating optimal nose proportions, rather than to test the system on a broader population or evaluate its clinical effectiveness. Informed consent was obtained from the participant for this study.
Consent for publication
The pictured patient who was treated by the authors of this manuscript has given her consent for her images to be published for research purposes.
Copyright
© The Author(s) 2025.
REFERENCES
1. Chopan, M.; Samant, S.; Mast, B. A. Contemporary analysis of rhytidectomy using the tracking operations and outcomes for plastic surgeons database with 13,346 patients. Plast. Reconstr. Surg. 2020, 145, 1402-8.
2. Khansa, I.; Khansa, L.; Pearson, G. D. Patient satisfaction after rhinoplasty: a social media analysis. Aesthet. Surg. J. 2016, 36, NP1-5.
3. Ishii, L. E.; Tollefson, T. T.; Basura, G. J.; et al. Clinical practice guideline: improving nasal form and function after rhinoplasty. Otolaryngol. Head. Neck. Surg. 2017, 156, S1-30.
4. Korkmazov, M. Y.; Lengina, M. A.; Dubinets, I. D.; Kravchenko, A. Y.; Klepikov, S. V. Some immunological aspects of targeted therapy in polypous rhinosinusitis. Russ. J. Immunol. 2023, 26, 301-6.
5. Alfano, C.; Di Cristo, S. Historical overview of rhinoplasty. In: Scuderi N, Toth BA, editors. International Textbook Of Aesthetic Surgery. Berlin: Springer Berlin Heidelberg; 2016. pp. 585-9.
6. Zhang, Z.; Li, Y.; Guo, J.; Weng, D.; Liu, Y.; Wang, Y. Vision-tangible interactive display method for mixed and virtual reality: toward the human-centered editable reality. J. Soc. Info. Display. 2019, 27, 72-84.
7. Daniel, R. K.; Kosins, A. M. Current trends in preservation rhinoplasty. Aesthet. Surg. J. Open. Forum. 2020, 2, ojaa003.
8. Pribitkin, E.; Greywoode, J. D. Sonic rhinoplasty: innovative applications. Facial. Plast. Surg. 2013, 29, 127-32.
9. Lovice, D. B.; Mingrone, M. D.; Toriumi, D. M. Grafts amd implants in rhinoplasty and nasal reconstruction. Otolaryngol. Clin. North. Am. 1999, 32, 113-41.
10. Topsakal, O.; Sawyer, P.; Akinci, T. C.; Celikoyar, M. M. Algorithms to measure area and volume on 3D face models for facial surgeries. IEEE. Access. 2023, 11, 39577-85.
11. De Guzman, J. A.; Thilakarathna, K.; Seneviratne, A. Security and privacy approaches in mixed reality: a literature survey. ACM. Comput. Surv. 2019, 52, 1-37.
Cite This Article

How to Cite
Download Citation
Export Citation File:
Type of Import
Tips on Downloading Citation
Citation Manager File Format
Type of Import
Direct Import: When the Direct Import option is selected (the default state), a dialogue box will give you the option to Save or Open the downloaded citation data. Choosing Open will either launch your citation manager or give you a choice of applications with which to use the metadata. The Save option saves the file locally for later use.
Indirect Import: When the Indirect Import option is selected, the metadata is displayed and may be copied and pasted as needed.
About This Article
Copyright
Data & Comments
Data
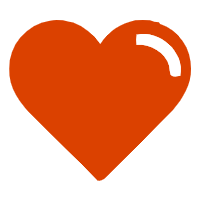
Comments
Comments must be written in English. Spam, offensive content, impersonation, and private information will not be permitted. If any comment is reported and identified as inappropriate content by OAE staff, the comment will be removed without notice. If you have any queries or need any help, please contact us at support@oaepublish.com.